Basic chatbots and conversational AI differ fundamentally in their technical capabilities, processing methods, and adaptability. You'll find basic chatbots operating on predefined rules with limited pattern matching, costing $5,000-$15,000 to implement. In contrast, conversational AI employs sophisticated NLP, contextual awareness, and machine learning algorithms, requiring $30,000-$250,000 investment. While basic chatbots handle simple queries, conversational AI excels in complex problem-solving and personalization. The following analysis reveals the transformative impact these differences will have by 2025.
Understanding Capabilities and Technical Architecture
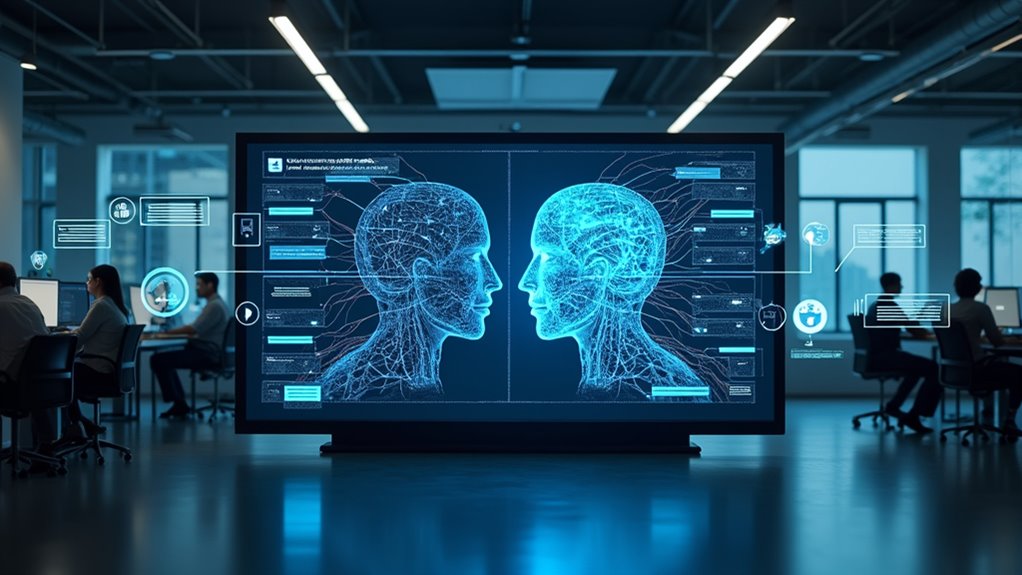
While both chatbots and conversational AI serve as automated communication tools, their underlying technical architectures differ substantially in complexity and capability. Basic chatbot frameworks operate on predefined rules and pattern matching, following linear decision trees with limited flexibility. You'll find they're restricted to specific use cases and struggle with context variations.
In contrast, conversational AI utilizes sophisticated AI algorithms, including natural language processing and machine learning models, to understand context, intent, and nuance. You're getting a system that can learn from interactions, adapt to new scenarios, and handle complex queries across multiple domains. The technical architecture incorporates neural networks, sentiment analysis, and contextual memory, enabling more natural, dynamic conversations that evolve beyond simple input-output patterns.
Natural Language Processing and Contextual Awareness
Because natural language processing capabilities vary greatly between these technologies, understanding their differences in contextual awareness becomes essential. While basic chatbots rely on pattern matching and predefined responses, conversational AI employs sophisticated semantic understanding to interpret complex user inputs. You'll find that basic chatbots often miss context from previous exchanges, whereas conversational AI maintains dialogue history to form coherent, contextually relevant responses.
The key distinction lies in how these systems process user intent. Basic chatbots identify keywords and match them to scripted responses, often missing nuanced meanings. In contrast, conversational AI analyzes linguistic structure, sentiment, and contextual cues to understand the true purpose of your query. This advanced processing enables conversational AI to handle ambiguity, maintain conversation flow, and provide more accurate, contextually appropriate responses across multiple turns of dialogue.
Learning and Adaptation Mechanisms
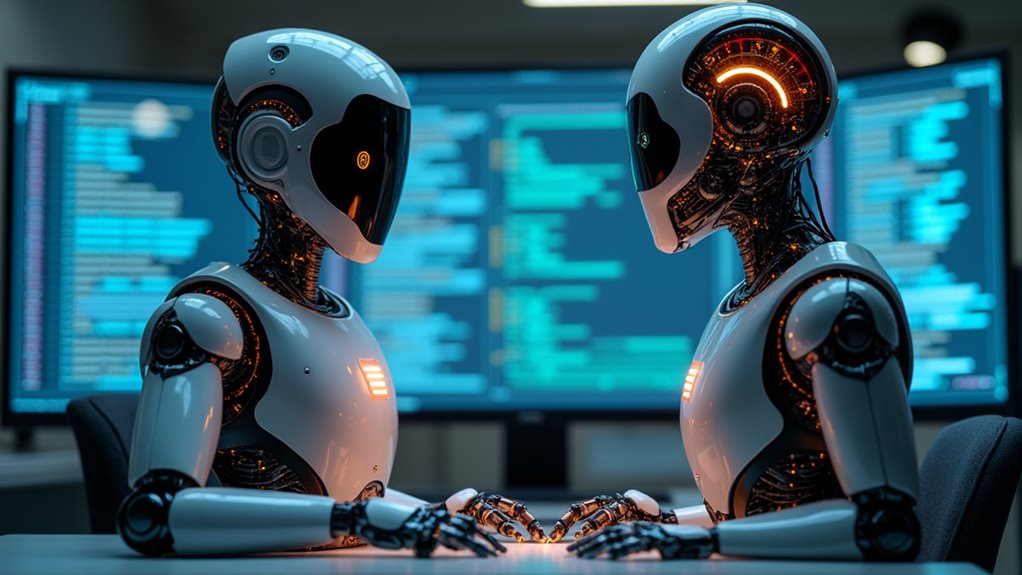
Since learning capabilities fundamentally differentiate these technologies, you'll find stark contrasts in how chatbots and conversational AI adapt to new information. Basic chatbots rely on predefined rules and remain static after deployment, requiring manual updates to expand their knowledge base.
In contrast, conversational AI employs sophisticated adaptive algorithms that continuously learn from each interaction. You'll notice how these systems analyze user feedback in real-time, adjusting their responses based on successful and unsuccessful exchanges. When you interact with conversational AI, it's constantly refining its understanding of context, tone, and user preferences. This dynamic learning process enables the system to improve accuracy over time, recognize emerging patterns in user behavior, and automatically incorporate new information without requiring extensive reprogramming.
Implementation Costs and Resource Requirements
The implementation costs between chatbots and conversational AI differ greatly in their initial investment requirements, with basic chatbots requiring minimal upfront capital while AI systems demand substantial infrastructure and development resources. You'll find that ongoing maintenance costs for chatbots remain relatively stable and predictable, whereas conversational AI systems require continuous optimization, model retraining, and potentially expensive computational resources. Your team's training requirements will vary considerably between the two technologies, as chatbot maintenance typically requires basic programming knowledge, while conversational AI systems demand expertise in machine learning, natural language processing, and complex system architecture.
Initial Setup Investment Comparison
Implementation costs represent a significant divergence point between basic chatbots and conversational AI systems, with stark differences in initial resource requirements and setup complexity.
You'll find that basic chatbots typically require a $5,000-$15,000 initial investment, primarily covering fundamental programming and basic interface development. The setup complexity remains relatively straightforward, often completed within 2-4 weeks using standard development frameworks.
In contrast, conversational AI demands a more substantial investment, ranging from $30,000-$250,000, depending on sophistication levels. This covers advanced NLP models, machine learning infrastructure, and extensive training datasets. While the initial cost benefit may seem to favor basic chatbots, conversational AI's superior capabilities and reduced long-term maintenance needs often justify the higher upfront expenditure. You'll need to allocate 3-6 months for proper implementation and training.
Ongoing Maintenance Cost Analysis
While initial setup costs represent a crucial investment factor, ongoing maintenance expenses reveal an even more significant long-term cost differential between chatbots and conversational AI systems. Your budget forecasting must account for distinct operational patterns and resource requirements.
- Basic chatbots typically require minimal maintenance costs ($500-2,000/month), focusing on script updates and basic troubleshooting, offering predictable cost efficiency for simple interactions.
- Conversational AI demands substantial ongoing investment ($5,000-15,000/month) for machine learning model refinement, data analysis, and constant optimization of natural language processing capabilities.
- You'll need to factor in specialized talent costs – chatbots require basic developers, while conversational AI systems need AI engineers, data scientists, and linguistics experts for maintenance.
These maintenance patterns directly impact your total cost of ownership and operational sustainability across platforms.
Team Training Requirements
Beyond maintenance considerations, team training represents a major cost driver in both chatbot and conversational AI deployments. You'll need to assess your team's current capabilities and plan for thorough skill development that aligns with your chosen solution's complexity.
Training Aspect | Basic Chatbots | Conversational AI |
---|---|---|
Duration | 1-2 weeks | 3-6 months |
Skill Level | Entry-level | Advanced technical |
Team Dynamics | Minimal collaboration | Cross-functional teams |
Cost Impact | Low-moderate | High-substantial |
Your training methodologies must adapt to each solution's unique requirements. While chatbot training focuses on basic configuration and rule-setting, conversational AI demands extensive knowledge in machine learning, natural language processing, and data analytics. Consider that conversational AI teams require ongoing education to keep pace with rapid technological advancements, whereas chatbot teams can typically maintain effectiveness with periodic refresher courses.
Real-World Applications and Use Cases
As organizations increasingly adopt digital transformation, both chatbots and conversational AI systems have found diverse applications across multiple sectors. You'll find distinct implementation patterns across industries, with each technology serving specific purposes.
- Customer service and retail automation lead the way, with basic chatbots handling standard inquiries and order tracking, while conversational AI manages complex problem-solving and personalized shopping recommendations.
- Healthcare solutions and financial services employ conversational AI for patient symptom analysis and investment advisory, whereas simple chatbots manage appointment scheduling and basic account inquiries.
- Travel assistance and education tools showcase the technology divide clearly – chatbots excel at booking confirmations and course registrations, while conversational AI provides personalized travel planning and adaptive learning experiences, often integrating with marketing strategies and entertainment engagement platforms.
Performance Metrics and Success Indicators
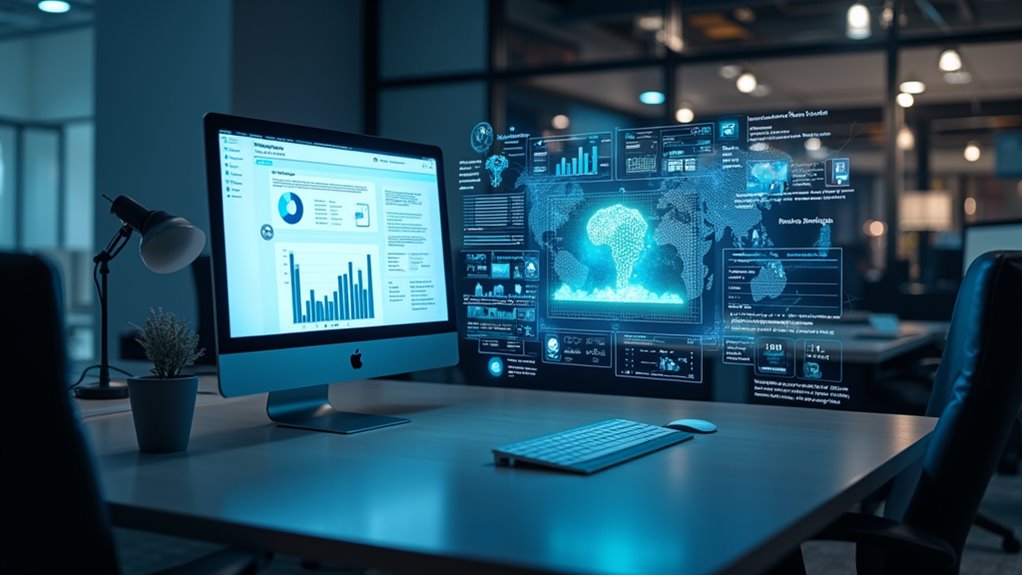
The effectiveness of chatbots and conversational AI implementations can be measured through distinct performance metrics that reflect their technical capabilities and business impact. You'll need to track specific performance evaluation metrics and success measurement indicators to gauge their effectiveness.
Metric Type | Basic Chatbots | Conversational AI |
---|---|---|
Response Time | < 2 seconds | 2-5 seconds |
Accuracy Rate | 70-85% | 90-98% |
User Satisfaction | 3.5/5 stars | 4.5/5 stars |
Resolution Rate | 60-75% | 85-95% |
Scalability | 100-500 concurrent users | 1000+ concurrent users |
These metrics provide quantifiable insights into system performance, helping you optimize your implementation and justify your investment. You'll notice that conversational AI typically outperforms basic chatbots across most performance indicators, particularly in accuracy and resolution rates.
Future Development and Integration Potential
Future platforms for chatbots and conversational AI will extend beyond current web and mobile interfaces, enabling you to deploy these solutions across emerging technologies like AR/VR, IoT devices, and wearable tech. You'll witness accelerated integration across diverse industries, from healthcare and finance to retail and manufacturing, as the technology adapts to sector-specific requirements and compliance standards. The cross-industry implementation will drive specialized development in areas like natural language processing, sentiment analysis, and context awareness, creating more sophisticated and adaptable AI communication systems.
Multiplatform Deployment Capabilities
While traditional chatbots often remain confined to single platforms, conversational AI systems demonstrate superior flexibility in cross-platform deployment and integration capabilities. You'll find that modern conversational AI utilizes multichannel support to maintain consistent interactions across diverse digital environments.
Consider these key deployment strategies that set conversational AI apart:
- Seamless integration across mobile apps, web platforms, and IoT devices with unified backend architecture
- Real-time synchronization of user context and conversation history between different channels
- Adaptive interface rendering that automatically optimizes for each platform's unique constraints and capabilities
You'll notice that this improved deployment flexibility enables organizations to maintain a cohesive brand voice and user experience, regardless of where customers choose to engage with the AI system.
Cross-Industry Growth Potential
Innovation in conversational AI continues to reshape industry boundaries, demonstrating exponential growth potential across multiple sectors like healthcare, finance, education, and retail. You'll find that while basic chatbots remain confined to specific industry applications, advanced conversational AI systems adapt seamlessly across numerous sectors through cross-industry innovation.
The market expansion potential for conversational AI greatly outpaces traditional chatbots, as these sophisticated systems can learn from varied datasets and apply insights across different business contexts. You'll see this technology evolve to handle complex interactions in medical diagnostics, financial advisory services, and personalized education simultaneously. This versatility enables organizations to utilize a single AI infrastructure for multiple purposes, reducing implementation costs while maximizing return on investment across different business units and market segments.