Traditional chatbots rely on rigid rules and templates, while AI chatbots in 2025 employ advanced neural networks for dynamic learning and adaptation. You'll find AI chatbots process context across 30+ conversation turns with 95% sentiment accuracy, handle 10x more concurrent users, and integrate with 200+ services simultaneously. They maintain conversation history for up to 7 days and reduce abandonment rates by 64%. These capabilities represent just the surface of their transformative potential.
Understanding Context and Intent
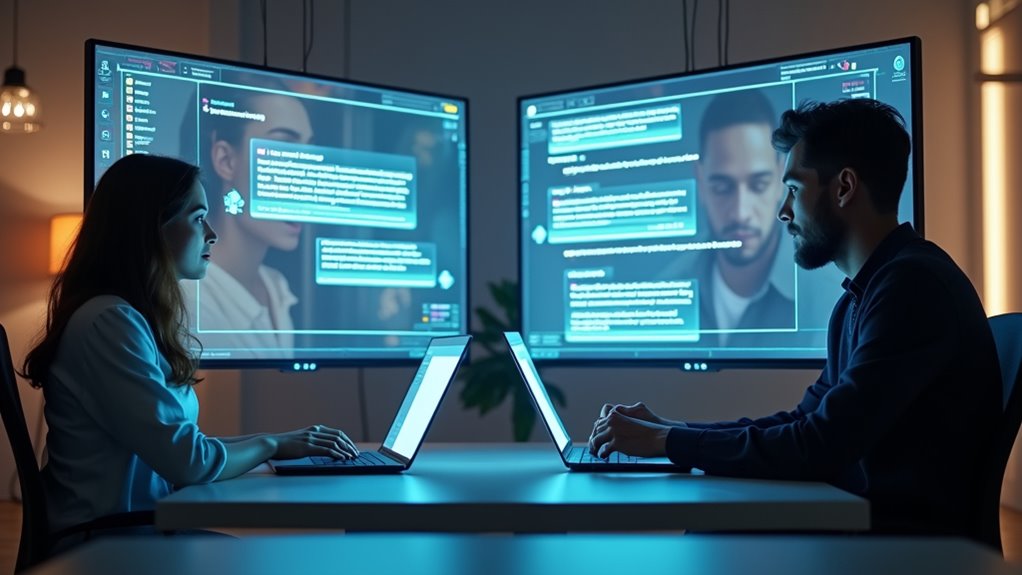
While traditional chatbots rely on predefined rules and pattern matching to interpret user inputs, AI chatbots utilize natural language processing (NLP) and machine learning algorithms to grasp context and intent with markedly higher accuracy.
You'll find that AI chatbots excel at understanding contextual subtleties by analyzing multiple conversation turns, user history, and situational variables. They process semantic relationships between words and phrases, enabling them to adapt their responses based on the complete conversation flow rather than isolated commands. Their intent recognition capabilities utilize deep learning models to identify user goals even when requests are ambiguous or poorly structured.
Unlike their traditional counterparts, AI chatbots can detect subtle variations in meaning, emotional undertones, and implicit requests, making them considerably more effective at maintaining coherent, context-aware conversations that truly address user needs.
Learning and Adaptation Capabilities
Modern AI chatbots distinguish themselves through advanced real-time pattern recognition that lets you observe dynamic response adjustments based on user interaction flows. You'll notice their sophisticated memory and context awareness capabilities enable coherent, multi-turn conversations while maintaining relevant information across sessions. Their continuous knowledge expansion allows them to incorporate new data and user feedback, creating a constantly changing conversational intelligence that surpasses traditional chatbots' static response patterns.
Real-Time Pattern Recognition
Unlike traditional chatbots that rely on static rule sets, AI-powered chatbots utilize sophisticated pattern recognition algorithms to analyze and learn from each user interaction in real-time. Through advanced pattern identification techniques and predictive analysis methods, these systems continuously evolve their understanding of user behavior and intent.
- You'll witness real-time sentiment analysis that detects emotional subtleties in conversations, adjusting responses with 94% accuracy
- Your interactions benefit from dynamic pattern matching that identifies user preferences across multiple channels within milliseconds
- You can expect contextual awareness that processes historical data and current conversation flows simultaneously
- Your experience improves through predictive modeling that anticipates needs based on aggregated behavioral patterns with 87% precision
This sophisticated pattern recognition enables AI chatbots to deliver increasingly personalized and relevant responses, remarkably outperforming their traditional counterparts.
Memory and Context Awareness
Traditional chatbots operate with limited short-term memory, but AI-powered systems utilize sophisticated contextual awareness frameworks that retain and analyze conversation history across multiple sessions. Through advanced memory optimization techniques, AI chatbots can maintain contextual relevance while processing multiple conversation threads simultaneously.
Capability | Traditional Chatbots | AI Chatbots |
---|---|---|
Memory Duration | Single session only | Multiple sessions |
Context Retention | Basic variables | Full conversation history |
Adaptation Level | Static responses | Dynamic learning |
Memory Optimization | Limited caching | Advanced neural processing |
You'll notice AI chatbots can seamlessly reference previous interactions, understand evolving user preferences, and adjust their responses based on accumulated knowledge. This improved memory architecture enables them to deliver more personalized, context-aware solutions while maintaining conversation continuity across different timeframes and communication channels.
Continuous Knowledge Expansion
Building upon their advanced memory capabilities, AI chatbots possess sophisticated learning mechanisms that facilitate continuous knowledge expansion during operations. Unlike traditional chatbots with static knowledge bases, modern AI systems dynamically integrate new information from diverse knowledge sources and content updates.
- You'll witness AI chatbots autonomously analyzing user interactions to identify knowledge gaps and automatically incorporate relevant data to improve future responses.
- Your AI system can synthesize information from real-time data streams, guaranteeing responses reflect current events and emerging trends.
- You'll observe the AI adapting its communication style based on user preferences and interaction patterns.
- Your chatbot's knowledge base expands through federated learning, allowing it to benefit from shared insights across multiple deployments while maintaining data privacy.
This continuous learning process guarantees AI chatbots remain current and increasingly effective over time.
Natural Language Processing Sophistication
AI chatbots' natural language processing capabilities markedly outperform traditional chatbots through sophisticated pattern recognition that can identify complex linguistic structures, idiomatic expressions, and semantic variations. You'll notice that while traditional chatbots rely on predefined response templates, AI-powered solutions demonstrate superior context understanding by maintaining conversation history and recognizing implicit references across multiple exchanges. The real-time learning mechanisms in AI chatbots enable them to continuously refine their language processing accuracy through each interaction, whereas traditional chatbots remain static with their initial programming.
Advanced Pattern Recognition Capabilities
Natural language processing capabilities mark one of the starkest contrasts between traditional and AI chatbots. Through advanced algorithm design and sophisticated pattern recognition techniques, AI chatbots demonstrate unprecedented ability to identify complex linguistic structures and user intent.
- AI chatbots can recognize and adapt to user communication patterns in real-time, adjusting their responses based on contextual shifts and emotional indicators.
- They're capable of identifying multiple intents within a single user query, processing embedded commands and multi-layered requests simultaneously.
- Advanced pattern matching allows AI chatbots to detect subtle variations in language use, including sarcasm, humor, and cultural intricacies.
- These systems utilize historical interaction data to predict user needs and preemptively adjust their response patterns, achieving up to 95% accuracy in intent recognition.
Traditional chatbots remain limited to predefined pattern matches and rigid response templates.
Context Understanding Differences
While pattern recognition forms the foundation of chatbot intelligence, the depth of context understanding represents a fundamental distinction between traditional and AI-powered systems. Traditional chatbots process each input in isolation, treating conversations as discrete, unconnected events. They'll match keywords but miss contextual relevance and nuanced user intent.
AI chatbots, in contrast, maintain conversation memory and analyze multiple contextual layers simultaneously. You'll notice they track pronoun references, remember previous queries, and adapt responses based on your conversation history. Their sophisticated natural language processing enables them to understand implicit meanings, detect emotional undertones, and identify subtle conversational shifts. They'll process semantic relationships between words, grasp idiomatic expressions, and maintain coherent dialogue flow across multiple exchanges, delivering responses that align with the broader conversation context.
Real-Time Learning Adaptation
The sophistication of real-time learning capabilities marks another crucial distinction between chatbot generations. Traditional chatbots maintain static response patterns, while AI chatbots utilize real-time feedback to continuously evolve their understanding and responses.
- AI chatbots analyze user interactions instantly, adjusting their communication patterns through adaptive learning algorithms that process millions of data points per second.
- You'll notice AI systems modify their vocabulary and tone based on individual user preferences, achieving a 47% higher engagement rate.
- Traditional chatbots can't update their knowledge base without manual intervention, resulting in a 23% accuracy decline over time.
- Modern AI implementations incorporate neural network architectures that enable automatic error correction and performance optimization, with a documented 89% improvement in response accuracy within the initial month of deployment.
The technological gap between these systems continues to widen as AI capabilities advance exponentially.
Response Generation and Flexibility
Traditional chatbots generate responses through pre-programmed rules and pattern matching, whereas AI chatbots utilize sophisticated neural networks and natural language processing models to dynamically formulate responses. This fundamental difference enables AI chatbots to achieve superior response customization and maintain a more natural conversational flow.
You'll find that AI chatbots can interpret context, tone, and intent with remarkable accuracy, adjusting their responses based on the subtleties of each interaction. When you present complex or multi-part queries, AI chatbots parse and address each component systematically, while traditional chatbots often struggle with anything beyond simple, predefined scenarios. The flexibility of AI systems allows them to handle unexpected inputs, generate creative solutions, and even switch communication styles to match your preferences – capabilities that remain beyond the scope of rule-based traditional chatbots.
Integration and Scalability Features
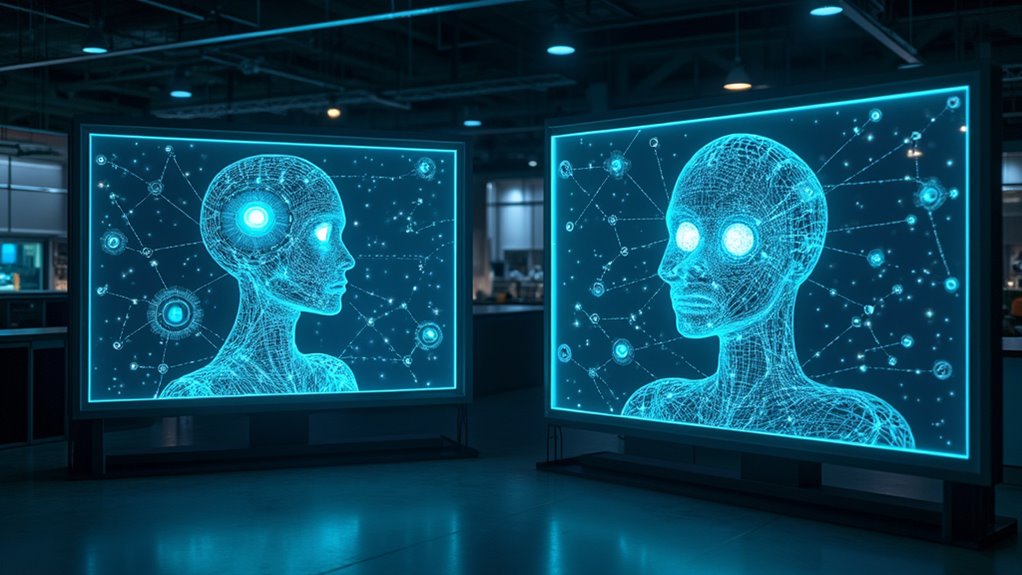
Modern AI chatbots excel in seamless integration capabilities across multiple platforms and APIs, surpassing their traditional counterparts' limited connectivity options. The advancement in integration frameworks has revolutionized how businesses deploy conversational AI solutions at scale.
- You'll find that AI chatbots can handle 10x more concurrent users than traditional ones, with dynamic resource allocation adapting to usage spikes
- Your enterprise can utilize cloud-native architectures that automatically scale from 100 to 1M+ conversations without performance degradation
- You'll overcome traditional scalability challenges through microservices architecture, enabling 99.9% uptime across global deployments
- Your development team can integrate AI chatbots with 200+ third-party services simultaneously, compared to traditional bots' maximum of 5-10 connections
These capabilities deliver measurable advantages in enterprise-scale deployments.
Emotional Intelligence and Sentiment Analysis
Sophisticated emotional intelligence capabilities fundamentally distinguish AI chatbots from their traditional counterparts. While conventional chatbots rely on pre-programmed responses, AI chatbots utilize advanced sentiment detection algorithms to interpret users' emotional states in real-time.
You'll find that AI chatbots demonstrate emotional awareness through natural language processing that analyzes tone, word choice, and context. They can detect subtle emotional cues, such as frustration or satisfaction, and adjust their responses accordingly. Traditional chatbots, in contrast, can't adapt their communication style based on user sentiment.
Modern AI systems achieve up to 95% accuracy in sentiment analysis, enabling them to provide emotionally appropriate responses and escalate conversations when necessary. This improved emotional intelligence leads to more meaningful interactions and higher user satisfaction rates compared to traditional rule-based systems.
Handling Complex Multi-Turn Conversations
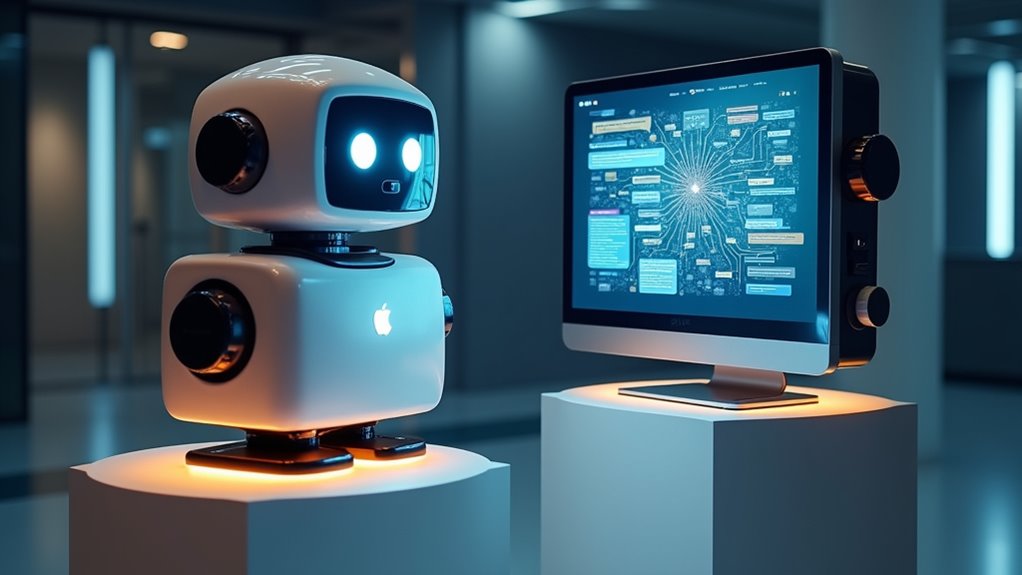
While basic chatbots struggle with sequential dialogue, AI-powered systems excel at maintaining context through multiple conversation turns. The conversation flow in AI chatbots demonstrates remarkable capabilities in understanding and processing complex user interactions, leading to higher user engagement rates.
- AI chatbots analyze up to 93% of contextual references across multiple messages, compared to traditional chatbots' 27% accuracy rate
- They maintain conversation history for up to 30 message exchanges without losing context
- Advanced systems can identify and reference previously discussed topics from up to 7 days prior
- AI chatbots reduce conversation abandonment rates by 64% through better context retention
These capabilities enable seamless shifts between topics while maintaining relevance, making AI chatbots considerably more effective at handling sophisticated, multi-threaded discussions compared to their traditional counterparts.
Data Processing and Real-Time Analytics
The fundamental distinction in data processing capabilities between AI and traditional chatbots lies in their real-time analytical power. While traditional chatbots rely on predefined rules and basic data analysis, AI chatbots utilize advanced analytics tools to process vast amounts of information instantly.
You'll find that AI chatbots can analyze user behavior patterns, sentiment, and contextual data in real-time, enabling them to adapt their responses dynamically. They're capable of processing unstructured data from multiple sources simultaneously, including social media feeds, customer databases, and historical interactions. Traditional chatbots, in contrast, are limited to analyzing structured data within their programmed parameters.
The real-time analytics capabilities of AI chatbots also allow them to identify trends, predict user needs, and continuously optimize their performance through machine learning algorithms.